Are Artificial Intelligence and Predictive Control Connected?
TRANSCRIPT
David Greenfield
Welcome to the Automation World Gets Your questions Answered podcast where we connect with industry experts to get the answers you need about industrial automation technologies. I'm David Greenfield, editor in chief at automation World. And the question will be answering in this episode is are artificial intelligence and predictive control connected now?
Joining me to answer this question is Bruce Slusser, the digital transformation practice director was system integrator Avanceon. So thanks for joining me today. Bruce.
Bruce Slusser
Thanks for having me. I appreciate it, David.
David Greenfield
So you know Bruce, you know, so that we're all on the same page with this potentially complex topic. Can you start by giving us a brief overview of what analytics and model predictive control are in the context of industrial manufacturing, of course.
Bruce Slusser Absolutely. I'd be happy too. So analytics refers to the process of collecting, processing, analyzing and interpreting large volumes of data to gain insights and make informed decisions.
In industrial manufacturing, analytics involves utilizing various data sources such as sensor readings, production data, quality metrics and more.
Basically just to extract meaningful patterns, trends and correlations about the data, these insights can help manufacturers identify areas for improvement, diagnose reoccurring issues, and make data driven decisions that can lead to better operational efficiency, reduce downtime, and improved product quality when we talk about analytics and all predictive control, there's several different types of analytics used in industrial manufacturing.
These typically include descriptive analytics where this is the process of summarizing historical data to understand what has happened in the past.
Diagnostic analytics is more about analyzing data to determine why certain events or outcomes have already occurred, and then we have predictive analytics.
And this is what we typically are using when we talk about model predictive control and our algorithms that that tie that together. And this is using historical data to forecast future events or trends that are going to happen based on real time data that's coming in and then last but not least, we have what is called prescriptive analytics.
Prescriptive analytics is recommending actions to optimize the outcomes based on analysis of current and historical data.
So you can think of predictive analytics as preprocessing and prescriptive as post processing. Model predictive control is really an advanced control strategy used in industrial manufacturing to optimize process performance by predicting future behavior and making control decisions accordingly. It involves using mathematical models that describe the dynamic behavior of the manufacturing process.
These models take into account various factors such as process variables, constraints and disturbances, model predictive control through the means of a feedback loop is typically what we're gonna be leveraging and the systems current state and set point are used to predict the future behavior that the process using that mathematical model is going to leverage. Different control actions are calculated to achieve the desired outcomes while satisfying operational constraints that we're working within and the control action that optimizes the process. The process is being continually monitored and the control calculations are updated based on real time data and those predictions in the model. So model predictive control is particularly useful in scenarios where processes are complex, nonlinear, and subject to various different types of constraints. It allows manufacturers to achieve optimal process performance while considering real time data and minimizing deviations from desired targets.
And really, in summary, analytics involves extracting insights from data to improve decision making and operational efficiency in industrial manufacturing model print of control is a control strategy and it uses those mathematical models and real time data to optimize those processes by making calculated control decisions to achieve the desired outcomes while adhering to those constraints that we talked about. Together, these concepts contribute to really a more effective and efficient manufacturing operation when used collaboratively.
David Greenfield
Well, wow, I'm glad we started off with that because there's not just one or two types of analytics that we're talking about here, but actually a number of them. And like you said, model predictive control is a strategy for applying that type of analytics. So thank you for explaining that. That does help kind of lay the groundwork for this discussion, for sure.
So, you know, given that the description of the different analytic types that you gave there, can you talk more about the specific benefits that analytics and model predictive control bring to the table for manufacturers? And are there any real world applications that stand out for you based on your experience working with manufacturers using these types of analytics?
Bruce Slusser
Sure. So both analytics and model predictive control offer significant benefits to industrial manufacturing sector really by enhancing that efficiency and quality and reducing cost that we talked about earlier on and some of the benefits of analytics and model predictive control in industrial manufacturing are we could start with discussing data, data driven decision making and this is also referred to as data storytelling through analytics and enables manufacturers to make informed decisions based on data insights. Really, this is gonna be leading to a better resource allocation, process optimization and strategic planning that we can also talk a little bit about process optimization.
So by analyzing historical and real time data, manufacturers can identify things like bottlenecks, inefficiencies, and opportunities for improvement within their process. By analyzing those patterns through the anomalies of their assets, model predictive controls, predictive nature in and of itself, helps prevent undesirable oscillations and control deviations for anticipating future process behavior and acting accordingly. And you know, lastly another benefit that we have is around energy efficiency. So this is really another growing use case for analytics and predictive control in analyzing energy consumption patterns. In doing so, manufacturers can identify ways to reduce energy waste and implement energy efficient practices. So after analyzing energy consumptions, model predictive control can begin by leveraging to optimize those control inputs, minimizing energy consumption and achieve energy efficient operation.
There are really many different real world examples of where model predictive control can be used in discrete manufacturing. So let's take a baking process, for instance, in a commercial bakery as an example. Baking involves precise control over temperature, humidity and time to really produce a consistent and high quality product model. Predictive control can be used to optimize the baking process in a bakery through a couple of different factors. And So what we've most typically seen is around temperature and energy. So temperature and humidity control in our bakery controlling the oven temperature and the humidity is essential to achieve uniform baking. You know, getting the desired texture and color in a very consistent manner with outside environmental factors, even inside of a manufacturing facility, are things that tend to disrupt the quality of those products.
And so as external parameters change, those conditions have an effect on the efficiency and the operation of those ovens. Model predictive control uses real time measurements and a you know a A process model to adjust these variables to maintain optimal conditions throughout a baking cycle, and we'll get into a little bit of how that deviates from a typical PID controller as well.
And our other area where we can, we can get a lot of gains is and we just talked about this as well, is energy efficiency. Pperative control can optimize those oven settings to reduce energy consumption while maintaining the product's quality. Oftentimes, again, this has to do with the different variables and the sensors that we're bringing in, rather than just running that oven at it's it's optimal prime power consumption. We can vary that power still knowing that our product is going to have a consistent quality and be able to predict that quality based on those variables that we're taking in and passing through our model. So it can determine the optimal time to switch off heating elements or adjust the temperature based on the products characteristics.
You know, this may take into effect different vision systems that we're seeing for for that ensure that the right, the right brownness of our bread for instance is one of our variables and really this is very different from just a standard heat controller.
So taking our our example from above, using model predictive control in the baking process offers several advantages over our traditional PID controller. While both approaches will aim to regulate the temperature and other process variables, they really differ in their methods and, most importantly, probably they're capabilities.
So let's kind of just talk a little bit about how we can compare those two different approaches side by side. So a traditional PID heat controller is it uses a combination of proportional integral and derivative terms to compute the control output. It reacts to the different the difference between the desired set point and the current process variable. To adjust the control action that comes out of that PID controller, PID controllers require manual tuning of both of those three different terms. So the PI and the D to achieve really a stable and responsive control, uh tuning can be a very time consuming and not always result in optimal performance. Oftentimes, the tuned loop can become inefficient over time just due to equipment degradation since it was tuned when the add equipment was brand new, working at its optimal efficiency out of the box. If you will, and so you know PID controllers are really designed to maintain stability around the set point, but they might struggle with handling complex, nonlinear or time varying processes.
Traditional PID controllers are typically designed for a single input, so this is known as single input, single output control. So in our bakery example this might mean using multiple PID controllers for different process variables of in temperature might have a controller. Humidity might have a controller. Different units of measure. Each one of those having different controllers and control points and that can lead to some challenges in coordinating those control actions together. They may work very well independently one from another, but as you try to bring those variables together, it could be very challenging.
And so lastly PID controllers react to the current error. This is our deviation from our set point without really considering any future process behavior.
Many control engineers are going to know that the derivative portion of the controller is somewhat that futuristic piece, right? But really it's a fixed point that we're trying to tune and that lack of prediction can lead to what we see as overshooting oscillations or slow response to any disturbances in the process. Taking a step into model predictive control, we can kind of see how these challenges with its standard PID controller can be overcome and so model predictive control really uses a mathematical model of the process and the dynamics to predict how the process is going to evolve in the future. It can then calculate control actions by optimizing a cost function essentially that considers future predictions and control constraints.
Modell predictive control optimizes control actions over a future prediction horizon, if you will, making the decisions that minimize the cost function while satisfying all the constraints that it's still working within, and this really enables model predictive control to handle complex nonlinear processes that we see in many manufacturing environments, it can.
It also handles multiple process variables and constraints that we talked about the multiple different types of units of measure above and really in thinking through that, that constraint model, predictive control eliminates all of those constraints really simultaneously. So allowing it to coordinate control across different types of variables and this is really beneficial in our baking example where temperature and humidity need to be controlled together in order to get optimal results, not independently model pit of control. Really also can predict how the process is going to behave in the future and calculates control actions accordingly and respectfully to how we have seen those variables change and those disturbances change in the past. So this predictive capability helps prevent that overshooting or optimize this transitions between the process phase and response to disturbances much more effectively. And so really, in the context of our baking process control model, predictive control offers advantages by taking into account the dynamic nature of the baking process, coordinating control actions across multiple variables and optimizing control actions based on future predictions.
While PID controllers, they certainly have their place and are more simpler to implement, but model predictive control is better suited for complex multi variable processes where optimal performance and adaptability is gonna be critical.
David Greenfield
That really shows how that this isn't just a technology for oil and gas and chemicals, which, while they have these highly complex processes that are of course is a great fit for, like you said, it could be used for baking. And the energy efficiency examples that you gave there really speak across industry, as that’s such a huge focus for industry overall now. So given that wide applicability of these analytics and model predictive control, in particular, what are the key elements that industrial manufacturing companies should have in place to really be able to leverage these technologies?
Bruce Slusser
Yeah, it's really, really important. So everything that we're gonna be doing depends on accurate data and robust data. So first and foremost, a robust data collection infrastructure is gonna be crucial. And this really involves having the proper sensors, data acquisition systems and connectivity in place to capture that relevant data.
So, you know, we'll start with focusing on our controls from data infrastructure 1st and and with this, we're gonna want to invest in getting our assets connected really by designing and implementing, implementing a control architecture that integrates real time process data capable of connecting to a high speed historical repository or historian service. You're gonna wanna look at how we can implement sensors. These can be IoT devices to collect relevant process data as well. And by relevant, I really mean ensuring that that data is gonna be very accurate and consistent with its with real time availability as well. Set up a robust data storage system that can handle large volumes of data and provide easy retrieval and access to that information.
This is, you know, really typical in the form of an industrial historian that we usually would see stood up in an on Prem manufacturing environment in more recent architectural designs as enterprises are moving towards a smaller infrastructure footprint, a cloud repository that can feed to a data warehouse or data Lake is also another really good option.
And then thinking about our oven example that we used, uh, we won't just want data that's directly connected to the asset being controlled, but we really wanna also ensure that you're integrating data from various sources. So this is including different process stages and systems as well to feed that comprehensive view of the production process, because taking a step back from traditional control methodology of our PID controller, we're able to bring in all those different variables, so machines that are upstream or downstream from us can be providing us meaningful information. And so getting data from not just our single source, but being able to feed that data to our model from other assets is also gonna help that model generate a more accurate futuristic view of what's happening based on the live variables that are coming into it.
Beyond the the infrastructure, we can begin focusing on the analytics and model predictive control components themselves. So we've got our data infrastructure, we've got a lot of real time data streaming. We've got a good historical data source and now we want to deploy the appropriate analytics tools and software capable of processing, analyzing and visualizing that collected data.
So let's look at, you know, we'll want to implement data processing techniques, and this is gonna be to really clean, filter and transform that raw data into usable formats for our analysis. This is most commonly referred to as ETL or extract, transform and load, and then lastly we want to develop predictive models using machine learning algorithms to forecast the process behavior kind of forecasting a little bit. Some of our AI discussions that we can get into and then detect potential issues and optimize those outcomes leveraging those models that we can start to incorporate.
And when we talk about model predictive control, there's a couple of different scenarios that we can get into as far as how you would think about rolling this out in a manufacturing environment. Industrial manufacturers today have PLC controllers with the capability of in a limited form being able to do model predictive control right with inside the controller. This is really good for point applications where you might not need scalability across a large process versus a standalone solution which is gonna leverage a potentially a server that you have on Prem where you would install the model predictive control software, the algorithms and the analytics tools on a separate server feeding information back to your controller and so that is really a fork in the road if you will. When you're working on deciding what type of solution I need based on scalability.
So from a capabilities perspective, they're both off you very good model control, but how scalable are you gonna need and thinking two years, three years down the road, what might come and how you can start to leverage it more or if you do have just a small application and that can help you it considerably when you're thinking about cost and budgets as well?
David Greenfield
So now, Bruce, you know, since you're the digital transformation practice director at Avanceon, I've gotta ask: Can model predictive control be integrated into a digital transformation plan and contribute to the, you know, the implementation of the Smart Factory initiative. And if it can, how?
Bruce Slusser Certainly model predictive control really does play a vital role in the digital transformation journey and align seamlessly with the vision of smart factories today. So integrating model predictive control strategically into your digital transformation plan involves leveraging its predictive capabilities to optimize complex manufacturing processes in real time that we really didn't have the capability of doing 20 years ago. And so, you know, imagine that as part of your smart factory initiative, you've already collected a wealth of real time data from all kinds of sensors throughout your plan. You've got all your machines connected and all of your production lines are feeding this data to your historical models.
Today, model predictive control is gonna take this data and combines it with dynamic processes that really models to make an accurate prediction out of your future process behavior. So by doing so, he anticipates how changes in variables are going to impact your your process and allows your your people to really focus more on not so much those individual inefficiencies, but taking a step back and seeing your entire plant as as one holistic living unit, if you will.
So model predictive control doesn't just stop at at prediction, it calculates optimal control actions to ensure the processes operate within desired constraints and goals. This means minimizing energy consumption, reducing waste, maintaining product quality, and really maximizing efficiency, which is all around some of our foundational elements of our digital transformation journey and where we get a lot of our ROI from outside of just focusing on on evolving and giving our people the tools that they need to become more efficient as well.
David Greenfield
I think we may have well covered this earlier, but I just wanna make sure because I've just been thinking about this as we've been talking here, you know we mentioned you know that model predictive control has been widely used in the process industries, especially oil and gas and chemical for decades now and you know, we talked about other you gave the baking example was a great example, but what about more discrete manufacturing examples? Can it be applied there as well, and if so, what is it typically used for? Is it more for energy efficiency as you mentioned earlier or are there other applications?
Bruce Slusser
Sure. I mean, as you mentioned it, it's done wonders in our process industries today. You know, we talked about complex processes that that exist and so model predictive control is definitely not limited to just the process industries alone as we've as we've shown it can definitely be applied in discrete manufacturing settings as well.
In fact, while it's origins are in the process, industries like you mentioned oil, gas, chemicals, model predictive control has found its way into various manufacturing sectors. You know, discrete manufacturing which involves producing individual items or units like lows of bread, or electronics anc automobiles as opposed to a continuous process like gas and oil, right? So in discrete manufacturing model, printed control is often used for optimizing production processes that involve complex sequencing. You know, resource allocation and maybe several different scheduling constraints that you're working with in the discrete manufacturing environment as well, taking into account different types of product changeovers and how equipment changes from developing.
Oftentimes, there's enough deviations in that processing side of things that causes some waste early on as that machine or sequence of machines ramps up and so model predictive control being able to take in those different variables going from one manufacturing product to the different manufacturing product allows that equipment to essentially warm up, if you will, right, in a in a more efficient manner. And so overall model, I'm sorry, model predictive control in discrete manufacturing enhances production efficiency and really allows us to reduce cost at several different levels.
So not just from that waste perspective where we have bad product being created for a certain period of time, being able to reduce that time from where we're manufacturing bad quality product. But as you mentioned, energy efficiency and being more sustainable are areas where discrete industrial manufacturing can really take advantage of model predictive control. And just allows us to be more adaptive with our production scheduling.
David Greenfield
Thanks for clarifying that. I know it was kind of expanding on our earlier discussion of it, but that kind of helped put it into perspective here across all the industries we cover here at automation world.
Bruce Slusser
Sure.
David Greenfield
Yeah, since, you know artificial intelligence it or at least discussions of it or everywhere now, if not the actual application of it, can you provide an example of how AI can be used in conjunction with analytics and model predictive control? Because I would seem that this would be a pretty ripe area for its use.
Bruce Slusser
Absolutely. And it's certainly in its infancy right now with manufacturing for sure being so new. And you know, there's there's different approaches to how you can implement AI. Yeah, let's kind of talk about an example really of how artificial intelligence is used in conjunction with analytics and model predictive control in the context of our smart manufacturing facility, for instance, so.
Imagine a a smart factory that's producing semiconductor chips. The goal is really to optimize that manufacturing process for efficiency, yield and quality. The same factors that we've been talking about earlier on, but you know, here's more how AI analytics and model predictive control can kind of work together. So as in our previous examples, we still begin with data collection. It always starts there.
It's important to remember that when dealing with AI, the larger our sample size for training the, the more accurate that our models are going to be, and this is really the the historical data that's used to analyze and identify patterns and correlations that AI leverages very heavily. And when we talk about that, we're really talking about machine learning models and these models are applied to predict potential issues or deviations in a manufacturing process. So, for example, AI algorithms can anticipate variations in chip quality based on historical data and those real time measurements that are coming in. The predictive models can identify subtle changes in process variables that might lead to defects or reduced yield.
So, for instance, AI can help identify if a change in the gas flow rate is going to affect chip quality. This prediction is really based on training models and historical data that has been configured to tell the model when a good or a bad chip has been produced. Based on that feedback, the machine learning model can better predict next time whether those current parameters are gonna yield a bad quality chip. And so in turn, allowing for that corrective action up front is gonna help us reduce any losses on that product, especially when it comes to a very expensive processing process.
So as we discussed a little bit earlier, model predictive control algorithms, they take in multiple variables, those variables can come from a machine learning algorithm that we've just touched on and the AI system is designed to adapt and learn from the ongoing manufacturing process as new data continues to feed that model and AI models are updated to account for changing conditions and process dynamics. This adaptability really ensures that the predictive insights remain accurate over time and and are still relevant even as the manufacturing environment and the equipment may lose efficiency over time.
So really in in this example a I would like to say is AI enhances predictive capabilities of our model predictive control by continuously updating and improving those process models, it helps the the smart factory maintain high quality production and efficiency and adapt to changing conditions, ultimately leading to an improved manufacturing outcome and reduced operational costs.
But at the end of the day, I do consider it as of right now in its current stage a way of us basically being able to enhance what model predictive control has already brought to the table by bringing in those different process variables and allowing that model to say this was a good manufactured chip and it happened with these conditions and this is a bad manufacturer chip. It happened under these conditions and that's happening every single time that it's that, it's that a unit is being made. It feeds that model and the more training data that we have, the better that that model will get over time.
David Greenfield
So you know, since the applications of any of these technologies that we're talking about, you know today or are mostly around more complex applications, even if it is a fairly simple production process like, you know, baking bread as you give you know. But given that that they're applied to more complex aspects of those production processes, what should users be aware of if they're looking to invest in related software or apply these AI technologies to their predictive control applications?
Bruce Slusser
Yeah, investing in software and applying AI technologies to predictive control applications can be a significant undertaking. So to make you know informed decisions, users should really be aware of of several different key considerations ahead of of starting down this journey. And so really like any successful project, before investing in AI software, we want to make sure that you're gonna just define clear objectives for your predictive control applications. Understand what you want to achieve, whether it's optimizing the process, whether you're trying to reduce costs, improving, you know product quality or enhancing safety, even any of those factors, we really wanna hone in on early on and make sure that we've got a very clear vision of what we're
Oftentimes it's misconstrued when talking about model predictive control in AI, the lines blur as to just you know, how much can I solve and what all is it gonna do and and kind of taking a step back from that and focusing on what it is that we just really want to improve on. Take one or two of those factors and build your your project around that need so you know assess the quality and the availability of the data for the systems or assets that you're looking to integrate.
We talked about high quality data is being really important to the success of these projects and so relevant data is gonna be crucial for building accurate predictive models, ensure that you have access to that sufficient historical data and still to real time data streams. Consider the trade off between model complexity and you know Interoperability. So when we talk about a complex AI model like deep learning and neural networks, they may offer very high accuracy, but they can be really challenging to interpret and explain.
They also could be costly to implement because it takes much more time to train those models. And so really choose a model that aligns with your specific needs, and what you're return on investment is gonna be. Ensure that the the software can integrate with your existing control systems.
You know, oftentimes with our data infrastructure and our HMI this when we start to look at software solutions that are out there, you've invested a lot of money in, in those systems that are at running your plans today. So make sure that you're you're aligning with a system that's gonna be able to integrate with the things that you have today and still meet your needs as you scale out in the future. Compatibility is going to be critical for a smooth implementation, so you know we're seeing many of these products leverage a a hybrid approach of cloud resources for modeling. And then the on-prem software for the controls pieces and so just make sure that you're keeping that in consideration with all of the other you know considerations, especially around when you talk about your digital transformation journey has a plant and a smart manufacturing facility—how that's gonna feed to future work and work streams as you go through upgrade and obsolescence projects and then assess the computational resources that are going to be required to run AI models effectively. Depending on the complexity of your application, you may need to invest in a high-performance computing resources. This could mean upgrading servers and things like that on Prem and this is another reason why we are seeing a lot of this processing being done through cloud solutions rather than maintaining hardware on site.
We also want to make sure that you're going to allocate enough time and resources for that model development. So there's the training and fine tuning of those models that we wanna do up front training IAM AI models can be, you know, intensive and does require specialized expertise. So you wanna set realistic timeframes and goals as well and then with all digital transformation initiatives we have to invest in our people. So invest in workforce development. Ensure that you have the necessary access to external expertise and your partners, data scientists, AI engineers and your controls engineers are definitely needed to be able to implement and maintain the AI driven predictive control.
And then lastly, we really want to evaluate whether the chosen software and the AI models can scale to accommodate, you know, are changing production volumes or process variations, model predictive control solutions can come in the form of both, you know, depending on depending on your, your architecture, your hybrid needs or a full on-prem solution. And so remembering that that scalability and flexibility are essential for really gonna, you know, you're adoption and you're evolving needs. So really, by just kind of carefully considering those factors, you can make informed decisions when investing in software and applying an AI technologies to implying your AI technologies to predictive control applications. Taking a a systematic approach and a well planned approach is gonna definitely help lead to a successful implementation and drive those operational improvements and competitive advantages.
David Greenfield
Now earlier we talked about the key elements that manufacturers need to have in place to use analytics and model predictive control, but what about some of the common challenges that you've seen manufacturers that they tend to encounter when they're actually working through the implementation of these technologies?
Bruce Slusser
Yeah, oftentimes model printed control and they are is blanketed as a a magic wand solution if you will, and it's implementation can come with various challenges. So developing accurate process models is a fundamental challenge in model predictive implementation. Real world processes can be complex, nonlinear, and subject to various different disturbances, so creating models that actually capture those dynamics and interactions accurately is crucial, and a challenge with model predictive control.
So several different challenges that could exist would could include things like gathering sufficient data to build the accurate models you may not have enough connected assets. You may not be at a point in your digital transformation journey where you've got the connectivity and signals in place yet so that foundational element that we had discussed maybe lacking. Choosing the appropriate model techniques for this specific process that we talked about deep learning models, which can be very complex and maybe far more advanced than what you would need to actually be able to even just get your ROI you're looking for. So making sure that you're you're managing those expectations and and meeting up with a model that's gonna be sufficient for your needs without investing too much time and and and and money and then dealing with uncertainties and changes in your your process behavior over time, ensuring that the model will remain valid as a as the process evolves. So keeping it up to date, you know you, you you change out equipment, you have a capital project where maybe you're doing some rework of your assets, making sure that you're going back and keeping those models up to date that they're not working without dated information or you know, a sensor that has been removed in some cases and it's trying to do things that it doesn't have a means of being able to compute.
So just having those components in place to keep things up to date and keep everything working tidy and then integrating model positive control into the existing control infrastructure. So you're communication networks and hardware can be complex and require specialized expertise. Well, these challenges can be daunting, but they're definitely not insurmountable, right? Successful model predictive control implementation requires careful planning, collaboration between cross functional teams, ongoing monitoring, and a willingness to adapt and learn from initial implementations. Piloting model puts of control on a small scale or using simulation environments before a full fledged deployment can help identify and address potential challenges early on in the process before you've invested too much down the road.
David Greenfield
So Bruce, I know earlier, you mentioned that we're still pretty much in the early days of applying AI to these analytics, but I'm going to ask you if you don't mind to put on your prognostication hat here for just a moment and you know where do you see these technologies heading in light of the way the application of AI is happening now?
Bruce Slusser
Absolutely. There's a wide future right now for AI in the manufacturing environment. And so we're likely to see more advanced AI and machine learning techniques integrated into our daily analytics and model predictive control systems. This is really going to enhance our predictive capabilities and enable adaptive control strategies and provide even more accurate insights for optimizing manufacturing operations.
I see these AI models being used more and more in uh, enhancing existing operations that we have today rather than replacing, you know we've talked a lot AI's been surrounded by a lot of controversy, good, bad and indifferent about replacing functions at at different levels, you know industries and you know jobs and and things of that nature, but really where AI gains its its most prominent uh ability in in our industrial environment is enhancing the things that we have today. This includes our analytics and our reporting features that we have.
So we have these slews of data that we're starting to collect with industry 4.0 and digital transformation initiatives and smart factories. We have the abilities to build interactive dashboards and analytics and reporting AI is going to give us the ability to feed those same data structures 2 models where we can ask in normal terms how well is my oven 6 operating in the last week in normal communication like that we can get data back and so we can now have a in lack of better terms, a conversation with our assets that is intuitive and doesn't require us to be data scientists ourselves. That's where I see the future going to where we're now able to do more self serving of our data rather than relying on our analysts and our data scientists have to go out. They can focus on their jobs of being able to extract and clean and feed our models, while now we can go and just ask in simple terms the information that we're looking for.
David Greenfield
Alright, so this has been a really a very targeted discussion, but a very broad one too. At the same time, with all we've been, we've always captured here in the discussion so far. So just have one last question for you, Bruce, and that is given the wide ranging aspects and possibilities for these technologies, could you share some key takeaways for our listeners who are looking to adopt analytics and model predictive control in particular?
Bruce Slusser
Absolutely. So when it comes to adopting analytics and model predictive control, there's several key takeaways that can guide your journey. First, remember the data is your greatest asset. Collecting, organizing and cleaning data is the cornerstone of effective analytics and control strategies. Starting small with pilot projects allows you to test the waters and understand the practical implications of these technologies deeply. Understanding your process is paramount. Accurate insights and models stem from a strong grasp of your process, dynamics variables, and the constraints that your equipment is working within.
Collaborative teams combining expertise and control engineering, data analytics, process engineering and IT bring a diverse group that are really their perspective and drive a well rounded solution.
Lastly, embrace in iterative approach and foster a culture of continuous learning to adapt and evolving trends really to gain adoption. We're going to begin with those clear goals that we defined early on.
Define your objectives clearly, whether it's optimizing efficiency, improving product quality or reducing costs so that you can direct your efforts more effectively. Start simply and gradually move to more advanced techniques. This applies to both analytics and model predictive control alike data quality. Again, it's gonna be paramount. So ensure the reliability integrity of your data to derive accurate insights and control actions.
Executive buy in is also another tough one, right, as these initiatives might require investments in technology and workforce training. So preparing your team for that change is gonna be vital. Adoption demands adjusting training and sometimes a shift in mindset. So, you know, demonstrating the value of analytics and model predictive control through a pilot project before full scale implementation can inspire confidence and decision makers alike.
Lastly, we wanna remember that the journey takes time and patience and perseverance are key. Adopting analytics and model predictive control is absolutely a transformative, transformative journey. And it's really about consistently seeking opportunities to optimize our processes and enhance efficiency and drive innovation.
So by starting small learning from our experiences and collecting across different disciplines, your company is gonna be able to unlock the full potential of these technologies and achieve substantial improvements in your operations. Remember that while challenges might arise, these can be overcome with the right approach and the dedication to learn and evolve.
David Greenfield
Well, thank you again for joining me for this podcast, Bruce, and thanks of course to all our listeners and please keep watching this space for more installments of Automation World Gets Your Questions Answered and remember you can find us online at automationworld.com to stay on top of the latest industrial automation technology insights, trends and news.
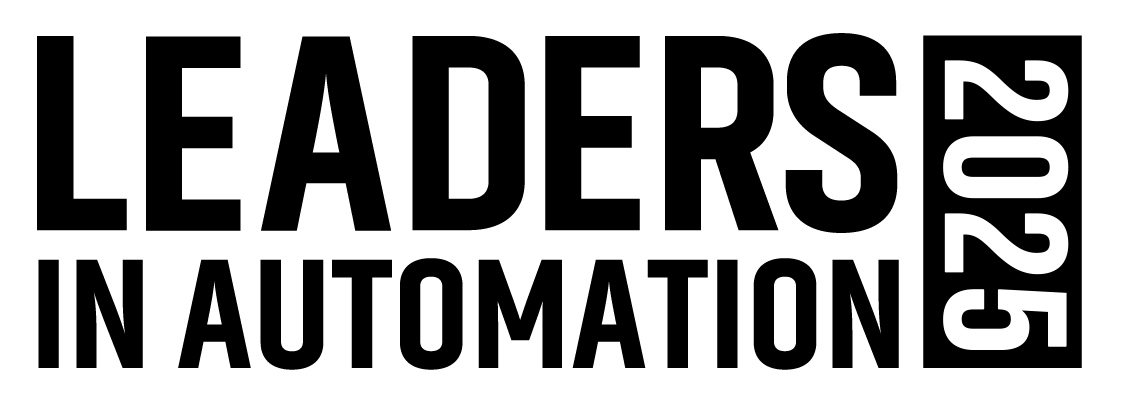
Leaders relevant to this content: