All the activity around Industry 4.0, the Industrial Internet of Things, and Digital Transformation is ultimately about one thing—analyzing large amounts of data to improve production and overall business operations. And while most discussions about Big Data and data analytics focus on overcoming obstacles associated with the aggregation and analysis of operations data, another challenge involves making the resulting analytics accessible and understandable to workers and management without the need for data scientists.
Self-service analytics (SSA) is designed to help businesses overcome this challenge.
According to technological research and consulting firm Gartner: Self-service analytics is a form of business intelligence (BI) in which line-of-business professionals are enabled and encouraged to perform queries and generate reports on their own, with nominal IT support. Self-service analytics is often characterized by simple-to-use BI tools with basic analytic capabilities and an underlying data model that has been simplified or scaled down for ease of understanding and straightforward data access.”
Applying SSA for continuous improvement
Lean Six Sigma is a common method employed by industrial manufacturing and processing companies for continuous improvement. Perhaps the biggest challenge in achieving success with Lean Six Sigma is getting everyone in the company actively involved in continuous improvement programs.
Petrosyan says these issues can be addressed when pre-work is completed by SMEs who can analyze the production data themselves and contribute to the central improvement projects or even reduce the number of projects by finding improvements on their own.
“Allowing process and asset specialists to contribute to these projects dramatically increases the operational improvements needed to meet the expected organizational goals,” he says. “By fully leveraging captured time-series data and more efficiently utilizing the operation expertise of process experts, companies establish a cornerstone to maximize organizational continuous improvement.”
Following are two case study examples provided by TrendMiner that showcase how SSA technology is being used to aid industrial continuous improvement programs.
Huntsman Corp.
A global manufacturer of specialty chemicals, Huntsman Corporation has been using analysis of time-series data as a key aspect of its digital transformation.
“For years, a Huntsman continuous isocyanate plant had been collecting and storing daily process and offline-created lab analysis data in a historian database,” explains Petroysan. “In 2016, the company’s teams used self-service analytics to build soft sensors based on operating conditions to predict product quality for certain isocyanates. Process experts used these sensors to make micro-adjustments to process setpoints to pro-actively minimize impurity levels. For example, one of the monitors predicted off-spec hydrolysable chloride levels in the final product. By adjusting vacuum pressure conditions, product quality was ensured. In addition, monitors were set up to send out early warnings alerting operators not to load trucks, thus preventing off-spec material from going out to a customer.”
With the aid of SSA, Huntsman process experts established 24/7 quality control compared to quality control with lab analyses that had previously only been available during regular weekday work hours.
“With trucks being sent out seven days a week, the soft sensors eliminated 75% of the expensive off-spec transportation cases which occurred on the weekends,” says Petroysan. “As a result, a significant positive impact on lead time was achieved as unnecessary wait hours for in-spec product were eliminated, with the average lead time being reduced by several hours. Finally, the extra insights into product quality also reduced the demand on lab resources as the number of uncertain situations for this specific product was reduced by as much as 10%.”
DSM Dyneema
Dyneema is an ultra-high molecular weight polyethylene invented and manufactured by DSM Dyneema, which claims Dyneema is the world's strongest fiber.
“By mapping the self-service industrial analytics functionalities to the different phases of the DMAIC cycle, it became clear that the tool could be applied to most of the phases and considerably reduce the time needed to complete each phase,” says Petroysan. “In a first test case, the define and measure phases were completed with self-service industrial analytics in two afternoons, instead of two weeks.”
Companies in this Article
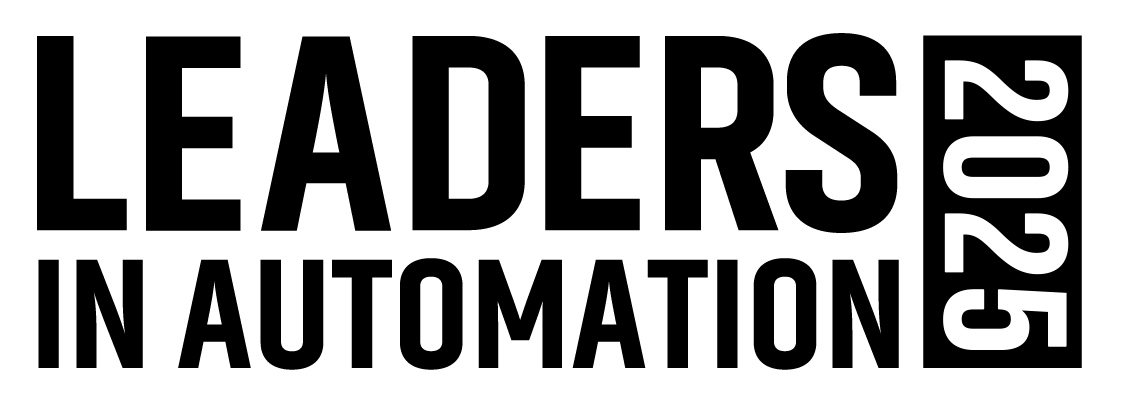
Leaders relevant to this article: